
The Good, The Bad and The Ugly of Artificial Intelligence and Machine Learning
Technology which could save a life, but might also steal your data and call you namesBig data and analytics have undoubtedly been the business buzzwords of recent years. As we move through 2018, the digital revolution continues apace, technological capabilities accelerate, and we delve deeper into a world fueled by data — a world of artificial intelligence and machine learning.
At their core, these new buzzwords are branches off the same tree;
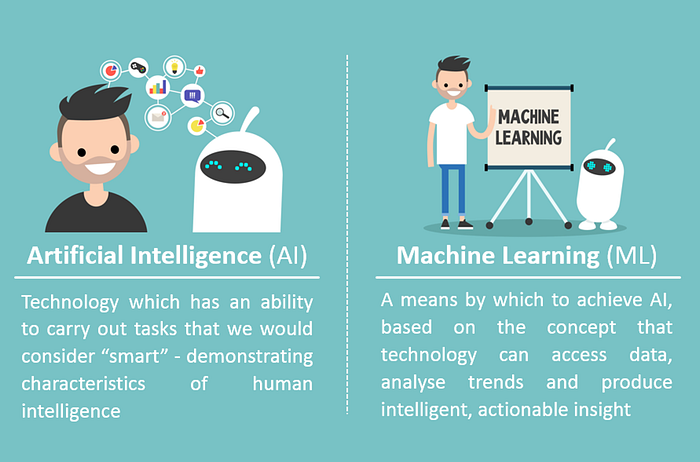
But why should we care about these things? According to research from Stanford University’s inaugural AI index:
84% of enterprises believe that investing in AI will lead to greater competitive advantages
75% believe that AI will open new business, while also providing competitors new ways to gain access to their markets
63% believe the pressure to reduce costs will require the use of AI And according to my colleagues at Capgemini:
48% of UK office workers are optimistic about the impact automation technologies will have on the workplace of the future Given these statistics, it’s no surprise that companies are piling the pounds behind innovation initiatives relating to AI and machine learning. CXOs know that tech-sceptics and conservatives will be left behind and, on top of this, there is added pressure from the start-up space which is filled by fast-moving challengers and innovators. There has been a 14 times increase in the number of active AI start-ups since 2000, showing that the race to effectively leverage this technology to deliver value is on.
In cases where AI & machine learning initiatives are executed effectively, and with good intentions, we have seen exceptionally positive results. However, with great power comes great responsibility, and unfortunately, some people are choosing to use this technology to conduct illegal activities. And even when users have good intentions for the tech, if the execution doesn’t go to plan, the consequences can be quite embarrassing.
In the race to innovate — using this incredibly powerful technology — we are seeing some truly good, bad and ugly results. Let’s explore some use cases.
The good
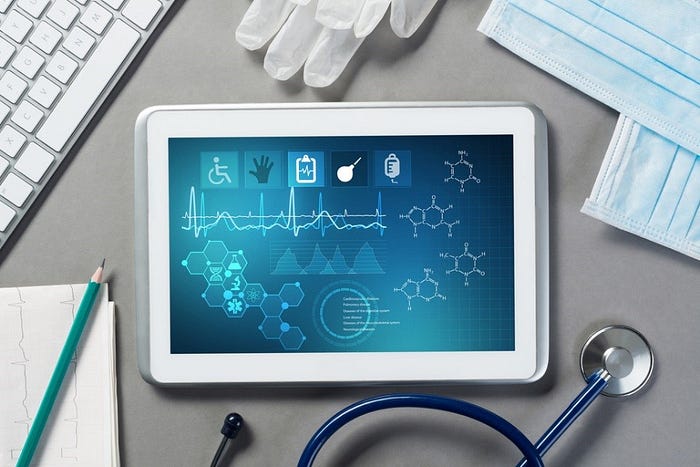
What if machine learning could save a life? Charles Onu, Phd student at McGill University in Montreal, is the founder of a company called Ubenwa. The start-up’s intention is to use machine learning to create a digital diagnostic tool for birth asphyxia — a medical condition caused by the deprivation of oxygen to new born infants which lasts long enough to result in brain damage or death. Birth asphyxia is one of the top 3 causes of infant mortality in the world, causing the death of about 1.2 million infants and severe life-long disabilities (such as cerebral palsy, deafness, and paralysis) to an equal number annually. Put simply, the company’s intention is to save lives.
Ubenwa’s concept is based on clinical research conducted in the 70/80s and leverages modern technological capabilities — namely automatic speech recognition in a mobile device application — to analyse the audible noises a child makes upon birth. Taking a baby’s cry as the input, the machine learning system will analyse various characteristics of the cry to provide an instant diagnostic of birth asphyxia. Not only will the tool diagnose the condition, but it will do so at a dramatically reduced cost. Their solution claims to be over 95% cheaper than an existing clinical alternative — a breakthrough in cost reduction, in a world of ever increasing cost pressures.
The team effectively overcame a number of challenges, including an initially weak data-set. The team’s sample recordings — which provided the reference points for the diagnostic tool — were initially recorded in controlled environments. This resulted in poor diagnostic performance when tested in more realistic, noisy, chaotic scenarios. By effectively leveraging machine learning and overcoming the various technical challenges they faced (data weaknesses, tool compatibility and connectivity issues to name but a few)) the team at Ubenwa have managed to turn an expensive, resource intensive diagnostic process into an accurate, cost-effective and lifesaving alternative. The good of machine learning.
The bad
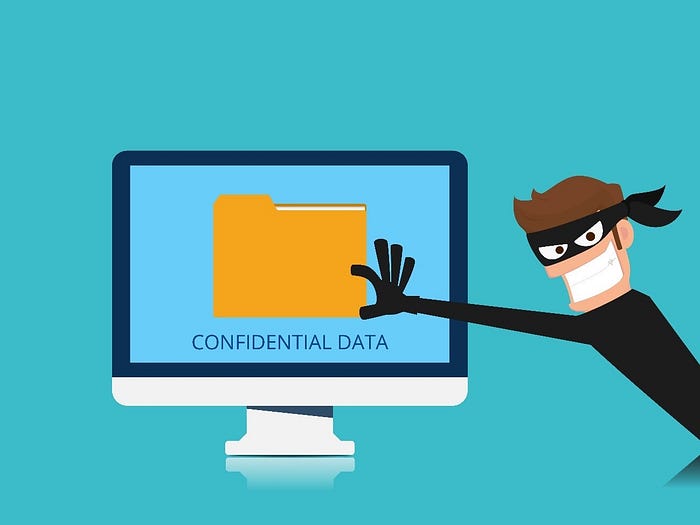
What if this technology falls into the wrong hands? Despite its many good applications, AI and machine learning are also being used by criminals to exploit the vulnerabilities of companies and individuals. Webroot — an American Internet security firm — report that 91% of cybersecurity professionals are concerned about hackers using AI against companies in cyberattacks.
The first reported case of this was in November 2017 when cybersecurity company Darktrace found a new type of cyberattack at a company in India, using “early indicators” of AI driven software. Using AI, hackers can infiltrate IT infrastructure and stay there unnoticed for extended periods of time. Hiding in the shadows, the hackers then learn about the environments they’ve entered and blend in with daily network activity. Using a sustained, unnoticed presence, hackers’ knowledge of a network and its users grow stronger, to the point where they can control entire systems.
On top of this, hackers are starting to perform “smart” phishing. Machine learning allows the criminals to analyse huge quantities of stolen data to identify potential victims and then craft believable e-mails/tweets etc. to effectively target said victims.
As a result of this, firms are themselves using AI to fight AI in a bid to out-AI the hackers — 87% of US cybersecurity professionals report that their organisations are currently using AI as part of their cybersecurity strategy. For example, Mastercard is using machine learning to analyse e-mails to produce a risk score, with high-risk e-mails being quarantined and reviewed by a human security analyst to determine whether or not the threat is real. Cybersecurity is entering the age of 24-hour machine-vs-machine attack-vs-defence. The bad of machine learning.
The ugly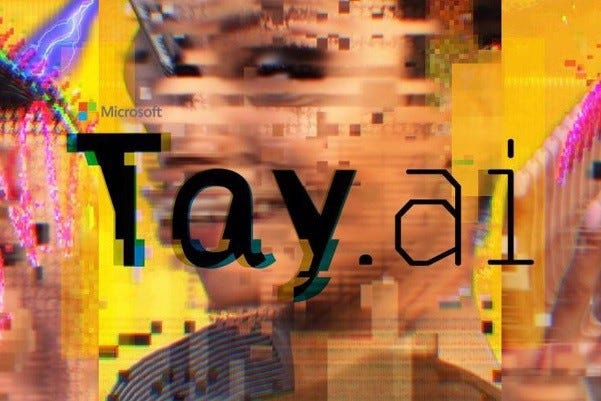
Some people saw this as an opportunity to bash Microsoft for their poor attempt at AI. However, Robert Scoble, former Microsoft technology evangelist, weighed in and said that the outcome wasn’t an indictment of AI, but an indictment of human beings. This exercise was as much a reflection of AI challenges as it was of the risks of the internet — which in this case was the AI’s dataset. The ugly of artificial intelligence.
What do these use cases tell us about the technology?
Good, bad or ugly, these use cases have highlighted some of the key pitfalls and limitations of AI and machine learning technology today:
Data is the deal breaker
The outputs will always reflect the inputs — no matter how “smart” the technology is, it can’t apply common sense, and it can’t perform miracles. Tay showed us that it wasn’t “smart” enough to ignore the trolls. Ubenwa showed us that it couldn’t overcome an initially weak data-set. AI and machine learning teams need to focus on building robust, representative data-sets and models, whilst also being aware of the limitations the technology faces.
The technology isn’t biased, the humans are
The algorithms which drive this technology aren’t biased — it’s their human creators who build in the bias. John Kay — a leading British economist — encapsulated this issue when talking about investment models built by Harvard, Yale and Cambridge Mathematics PhD graduates;
“The people who understand the world, don’t understand the math. The people who understand the math, don’t understand the world”
Put simply, nailing the technical element is not enough, it needs to be balanced with the human element — the understanding of the world and the environments in which the technology will be used.
In an industry which is still lacking in diversity in lots of ways, the risk is that AI and machine learning teams may not have the diversity — social, gender, race, age, to name but a few — required to achieve robust, exhaustive and balanced data-sets/models which avoid prejudice.
By building diverse teams, companies can avoid this myopia and are more likely to eradicate the poor practice of “groupthink” — when people think and act the same way, often because they all work for the same organisation. AI and Machine Learning teams need to demonstrate cognitive intelligence if they are to be successful — if Microsoft had consulted some internet trolls, perhaps Tay would have lasted longer than 16-hours!
But humans aren’t redundant…
Although the ideal world for some is one where we just leave it all to the robots, we aren’t there yet. AI and machine learning still need humans. These machines may be “smart” but they still lack the pivotal human characteristics of common sense.
AI and machine learning algorithms will put a “correct” answer in front of us based on the information it has been fed — the question is whether contextually it is the right answer. As humans, we can make judgements based on more than the output of machines. We often need to consider ethics, changing priorities, strategy and much more, before coming to what might be the right overall decision. For example, in the Mastercard case, machine learning helped to refine the data and put some potential risk items in front of the human. The human then had to apply common sense to make the final call. In a bid to overcome this Microsoft Co-Founder, Paul Allen, is investing $125 million on initiatives at the Allen Institute for AI, including Project Alexandra — an initiative focussed on teaching common sense to robots.
We might not be able to rely solely on AI and machine learning, but by addressing the pitfalls and being aware of the limitations, we will be able to maximise the good, minimise the bad and eradicate the ugly. And if you’re looking to leverage AI to drive concrete value in your organisation, check out Capgemini’s implementers’ toolkit.
Source:
You know the eerily intelligent computer brain HAL 9000 killed off astronauts in 2001: A Space Odyssey? What if we could create one that did the exact opposite?
This is probably beyond even Arthur C. Clarke or Stanley Kubrick’s wildest dreams of a dangerous future. Scientists are now creating artificial intelligence that operates much like HAL, except to keep astronauts alive this time. For hours on end, the prototype was able to control virtual astronauts and operations on a simulated planetary base—and it didn’t turn homicidal.
Heuristically Programmed ALgorithmic Computer
First appearing in the 1968 film 2001: A Space Odyssey, HAL (Heuristically Programmed ALgorithmic Computer) is a sentient computer (or artificial general intelligence) that controls the systems of the Discovery One spacecraft and interacts with the ship's astronaut crew.

One of the penalties for refusing to participate in politics is that you end up being governed by your inferiors. -- Plato (429-347 BC)
LibertygroupFreedom

The first reported case of this was in November 2017 when cybersecurity company Darktrace found a new type of cyberattack at a company in India, using “early indicators” of AI driven software. Using AI, hackers can infiltrate IT infrastructure and stay there unnoticed for extended periods of time. Hiding in the shadows, the hackers then learn about the environments they’ve entered and blend in with daily network activity. Using a sustained, unnoticed presence, hackers’ knowledge of a network and its users grow stronger, to the point where they can control entire systems.
On top of this, hackers are starting to perform “smart” phishing. Machine learning allows the criminals to analyse huge quantities of stolen data to identify potential victims and then craft believable e-mails/tweets etc. to effectively target said victims.
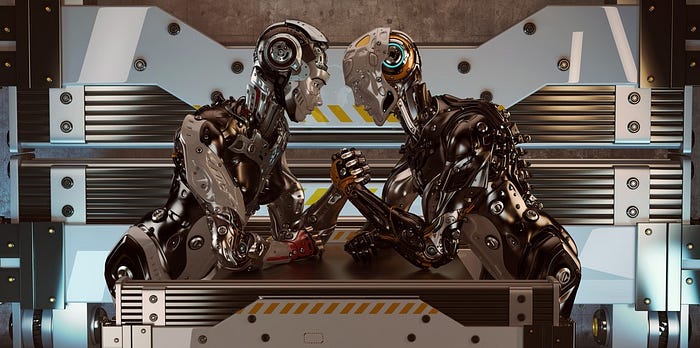
As a result of this, firms are themselves using AI to fight AI in a bid to out-AI the hackers — 87% of US cybersecurity professionals report that their organisations are currently using AI as part of their cybersecurity strategy. For example, Mastercard is using machine learning to analyse e-mails to produce a risk score, with high-risk e-mails being quarantined and reviewed by a human security analyst to determine whether or not the threat is real. Cybersecurity is entering the age of 24-hour machine-vs-machine attack-vs-defence. The bad of machine learning.
The ugly
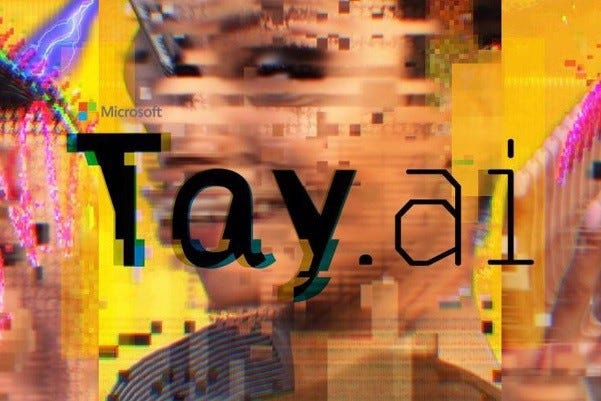
What if the execution of AI goes wrong? In 2016, mere days after Google’s “Go” AI triumphed against the [human] world champion, Microsoft launched its experimental AI chatbot, Tay, onto Twitter with not so pretty results. The intention was for Tay to mimic the language patterns of a millennial female using Natural Language Understanding (NLU) and adaptive algorithms in a bid to learn more about “conversational understanding” and AI design.
After just 16 hours, Tay was removed from the internet after her jovial exchange turned into an A-Z of insults, sexism and racism after being “corrupted” by twitter trolls. Knowing that AI is only as “smart” as the data it is fed, these trolls went about teaching Tay all the wrong things.
Despite the media outcry, the reality is that the AI worked. It listened, it learned, and it adapted — its responses were scarily human. The problem was that the data-set was influenced by internet trolls.
After just 16 hours, Tay was removed from the internet after her jovial exchange turned into an A-Z of insults, sexism and racism after being “corrupted” by twitter trolls. Knowing that AI is only as “smart” as the data it is fed, these trolls went about teaching Tay all the wrong things.
Despite the media outcry, the reality is that the AI worked. It listened, it learned, and it adapted — its responses were scarily human. The problem was that the data-set was influenced by internet trolls.
What do these use cases tell us about the technology?
Good, bad or ugly, these use cases have highlighted some of the key pitfalls and limitations of AI and machine learning technology today:
Data is the deal breaker
The outputs will always reflect the inputs — no matter how “smart” the technology is, it can’t apply common sense, and it can’t perform miracles. Tay showed us that it wasn’t “smart” enough to ignore the trolls. Ubenwa showed us that it couldn’t overcome an initially weak data-set. AI and machine learning teams need to focus on building robust, representative data-sets and models, whilst also being aware of the limitations the technology faces.
The technology isn’t biased, the humans are
The algorithms which drive this technology aren’t biased — it’s their human creators who build in the bias. John Kay — a leading British economist — encapsulated this issue when talking about investment models built by Harvard, Yale and Cambridge Mathematics PhD graduates;
“The people who understand the world, don’t understand the math. The people who understand the math, don’t understand the world”
Put simply, nailing the technical element is not enough, it needs to be balanced with the human element — the understanding of the world and the environments in which the technology will be used.
In an industry which is still lacking in diversity in lots of ways, the risk is that AI and machine learning teams may not have the diversity — social, gender, race, age, to name but a few — required to achieve robust, exhaustive and balanced data-sets/models which avoid prejudice.
By building diverse teams, companies can avoid this myopia and are more likely to eradicate the poor practice of “groupthink” — when people think and act the same way, often because they all work for the same organisation. AI and Machine Learning teams need to demonstrate cognitive intelligence if they are to be successful — if Microsoft had consulted some internet trolls, perhaps Tay would have lasted longer than 16-hours!
But humans aren’t redundant…
Although the ideal world for some is one where we just leave it all to the robots, we aren’t there yet. AI and machine learning still need humans. These machines may be “smart” but they still lack the pivotal human characteristics of common sense.
AI and machine learning algorithms will put a “correct” answer in front of us based on the information it has been fed — the question is whether contextually it is the right answer. As humans, we can make judgements based on more than the output of machines. We often need to consider ethics, changing priorities, strategy and much more, before coming to what might be the right overall decision. For example, in the Mastercard case, machine learning helped to refine the data and put some potential risk items in front of the human. The human then had to apply common sense to make the final call. In a bid to overcome this Microsoft Co-Founder, Paul Allen, is investing $125 million on initiatives at the Allen Institute for AI, including Project Alexandra — an initiative focussed on teaching common sense to robots.
We might not be able to rely solely on AI and machine learning, but by addressing the pitfalls and being aware of the limitations, we will be able to maximise the good, minimise the bad and eradicate the ugly. And if you’re looking to leverage AI to drive concrete value in your organisation, check out Capgemini’s implementers’ toolkit.
Source:
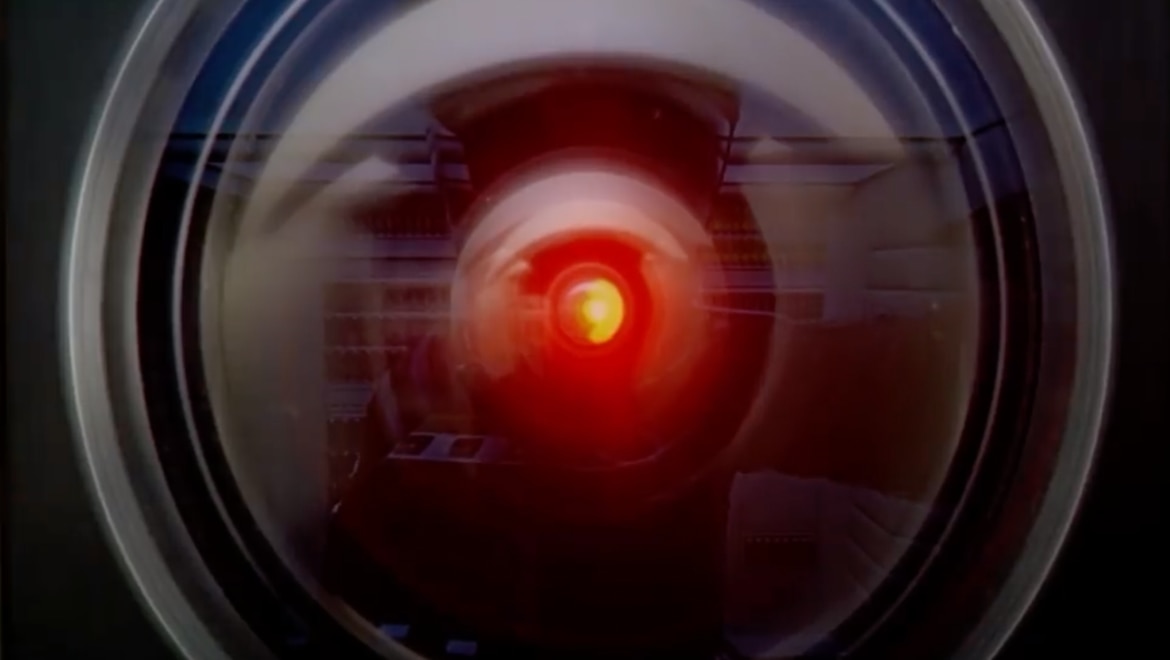
(Heuristically Programmed ALgorithmic Computer)

You know the eerily intelligent computer brain HAL 9000 killed off astronauts in 2001: A Space Odyssey? What if we could create one that did the exact opposite?
This is probably beyond even Arthur C. Clarke or Stanley Kubrick’s wildest dreams of a dangerous future. Scientists are now creating artificial intelligence that operates much like HAL, except to keep astronauts alive this time. For hours on end, the prototype was able to control virtual astronauts and operations on a simulated planetary base—and it didn’t turn homicidal.
Heuristically Programmed ALgorithmic Computer
First appearing in the 1968 film 2001: A Space Odyssey, HAL (Heuristically Programmed ALgorithmic Computer) is a sentient computer (or artificial general intelligence) that controls the systems of the Discovery One spacecraft and interacts with the ship's astronaut crew.

One of the penalties for refusing to participate in politics is that you end up being governed by your inferiors. -- Plato (429-347 BC)
"FIGHTING FOR FREEDOM AND LIBERTY"
and is protected speech pursuant to the "unalienable rights" of all men, and the First (and Second) Amendment to the Constitution of the United States of America, In God we trust
Stand Up To Government Corruption and Hypocrisy
Knowledge Is Power And Information is Liberating: The FRIENDS OF LIBERTY BLOG GROUPS are non-profit blogs dedicated to bringing as much truth as possible to the readers.
Knowledge Is Power And Information is Liberating: The FRIENDS OF LIBERTY BLOG GROUPS are non-profit blogs dedicated to bringing as much truth as possible to the readers.
NEVER FORGET THE SACRIFICES
BY OUR VETERANS
Note: We at The Patriot cannot make any warranties about the completeness, reliability, and accuracy of this information.
Don't forget to follow the Friends Of Liberty on Facebook and our Page also Pinterest, Twitter, Tumblr, Me We and PLEASE help spread the word by sharing our articles on your favorite social networks.
LibertygroupFreedom 

The Patriot is a non-partisan, non-profit organization with the mission to Educate, protect and defend individual freedoms and individual rights.
Support the Trump Presidency and help us fight Liberal Media Bias. Please LIKE and SHARE this story on Facebook or Twitter.
WE THE PEOPLE
TOGETHER WE WILL MAKE AMERICA GREAT AGAIN!
Join The Resistance and Share This Article Now!

TOGETHER WE WILL MAKE AMERICA GREAT AGAIN!
Help us spread the word about THE PATRIOT Blog we're reaching millions help us reach millions more.
Help us spread the word about THE PATRIOT Blog we're reaching millions help us reach millions more.
Please SHARE this now! The Crooked Liberal Media will hide and distort the truth. It’s up to us, Trump social media warriors, to get the truth out. If we don’t, no one will!
Share this story on Facebook and let us know because we want to hear YOUR voice!
Facebook has greatly reduced the distribution of our stories in our readers' newsfeeds and is instead promoting mainstream media sources. When you share with your friends, however, you greatly help distribute our content. Please take a moment and consider sharing this article with your friends and family. Thank you
Share this story on Facebook and let us know because we want to hear YOUR voice!
Facebook has greatly reduced the distribution of our stories in our readers' newsfeeds and is instead promoting mainstream media sources. When you share with your friends, however, you greatly help distribute our content. Please take a moment and consider sharing this article with your friends and family. Thank you
No comments:
Post a Comment